Jael santos and troy reyes aren’t your average bioengineers. You won’t find any fancy college diplomas adorning the walls of their home offices, let alone a high school degree—Santos and Reyes are teenagers.
The two are high school students in Los Angeles, California. When the afternoon bell rings at Benjamin Franklin Senior High School, they drive over to neighboring Caltech for sessions with EXPLORE, an externship program that connects local high schoolers with Caltech mentors for collaborative research projects. EXPLORE unites students with an array of scientific interests, and Santos’ passion is botany. She’s particularly inspired by the work of cacti researcher and Mexico’s first certified biologist, Helia Bravo-Hollis. But as Santos’ interest in botany blossomed, she began to see plant health as a tangible indicator of microscopic turmoil lurking in the soil.
“I thought, if plants need soil to grow, and soil needs the right microbes in order to work, and microbes need the right molecules as fuel—I just kept going smaller and smaller,” said Santos.
Within their externship program, Santos and Reyes are surrounded by like-minded, science-savvy students who are also compelled to investigate the unseen forces that influence environmental health. With help from their Caltech mentors, the EXPLORE research team hopes to solve a big problem brought on by tiny molecules: PFAS contamination in drinking water.
PFAS, or per- and polyfluoroalkyl substances, are synthetic compounds designed to make many consumer and industrial products nonstick, water-repellant, and heat-resistant. More commonly known by their infamous nickname “forever chemicals,” PFAS’ ubiquitous manufacturing use and near unbreakable carbon-fluorine bonds make them highly persistent in the environment and in our bodies. Some types of PFAS take up to thousands of years to decompose. And recent research has linked elevated PFAS exposure to increased incidence of thyroid disease, birth defects, and various types of cancer.
The EXPLORE team is developing a synthetic biology solution to solve PFAS’ synthetic chemistry problem. The idea: design a novel enzyme that binds and degrades PFAS, then scale up this enzymatic technology to remove PFAS from water supplies.
“There’s so much diversity in nature. We can only access a really tiny fraction of that right now. But we could, in theory, program all of that diversity,” said Alec Lourenço, a biochemistry and molecular biophysics PhD student at Caltech and this year’s EXPLORE mentor. Lourenço is developing a high-throughput platform that pools millions of data points on protein binding properties, making it simpler and more efficient for synthetic biologists to design better proteins.
According to Lourenço’s experience, modern protein selection is getting a major boost from generative AI algorithms.
“The more work we can do on a computer, the less we have to do in the lab,” said Lourenço.
Lourenço and his labmates use protein language models (PLMs) to predict protein shape, structure, and binding sites. Just as OpenAI’s large language model ChatGPT learns grammar rules by parsing human sentences, PLMs parse protein sequences to learn the grammar of life—the patterns in which proteins fold and interact with other compounds.
As our understanding of biology grows, the field needs tools that help scientists delve further into the unknown. These tools, like PLMs, already exist—but they only work if they’re intuitive for biologists to wield. The emergent generation of scientists—like Lourenço’s EXPLORE students—could be the trellis needed to support the continued merging of computer science and synthetic biology. While none of the EXPLORE students had machine learning experience prior to their externship, growing up alongside the advent of generative AI helps them see AI as a malleable tool rather than a disruptive force.
“I don’t think that AI is scary. I try to think of it as a way to extend what exists,” said EXPLORE team member Lucas Garcia.
The PFAS problem, as well as the larger field of synthetic biology, needs fresh perspectives from scientists more invested in exploring the unknown than staying in their field’s pre-defined lanes. Childlike curiosity, often stifled in markets that value production over experimentation, is hard to come by in today’s for-profit science sector. But in young scientists, that kind of curiosity flows like water.
The PFAS Problem
On April 10, 2024, the EPA released the first federal limits for PFAS in drinking water. Public water suppliers have until 2029 to prove that levels of six well-studied PFAS molecules are under ten parts per trillion. PFOA and PFOS, perhaps the most widely used “forever chemicals,” have an even more stringent cap of four parts per trillion.
“Four parts per trillion is like putting a couple of drops in the reflecting pond at the Lincoln Memorial,” said Frank Cassou, CEO of Cyclopure, a company that manufactures PFAS filters for industrial and at-home use. “It’s almost saying that PFAS can’t be present at all. So, you can imagine the kinds of technical decisions that people have to make to remove PFAS.”
Companies like Cyclopure produce filters that bind PFAS more precisely than existing methods. One method used by many public water suppliers is granular activated carbon, which adsorbs and traps organic compounds. Another effective method, anion exchange resins, uses positively charged beads to attract negatively charged PFAS. Both methods can remove up to 99% of PFAS under optimal conditions, but they don’t work as well on the smaller molecules, known as “short-chain” PFAS, and quickly lose effectiveness once their filters become saturated.
The EXPLORE team is developing a synthetic biology solution to solve PFAS’ synthetic chemistry problem.
Conventional physiochemical PFAS-filtering methods often fail to consider that the biological and environmental persistence of PFAS makes them more of a biology problem than a chemistry problem. And biology is messy.
When PFAS leach into water reservoirs, they become an infinitesimal component in a complex ecosystem. “In water treatment, there’s a lot of other things competing for filter adsorption,” said Cassou. Activated carbon and ion exchange resins don’t just bind PFAS—they can attract any particulates that interact with their chemistry. These filters can become saturated well before sufficient PFAS are removed from the water. This inadvertent concentration of organic matter is a feast for microorganisms, which flock to saturated filters and further contaminate the water by producing biofilm.
The EXPLORE team could circumvent this competition for filter space by forgoing a physical filter altogether. Instead, the team is designing enzymes that specifically bind and degrade PFAS. This synbio approach is more PFAS-precise than traditional treatments, but it requires a great deal of creativity to succeed. Synthetic biology empowers scientists to design molecules as they please, but what combinations of protein binding pockets, multi-layered structures, and amino acid configurations make the best PFAS-degrading enzymes? The sheer volume of viable options overwhelms human brains, but it’s nothing that a well-trained PLM can’t handle.
The EXPLORE team is using several PLMs in tandem to generate novel PFAS-degrading enzymes. First, they trained one PLM on datasets of naturally occurring enzymes that degrade molecules in different ways. Once the PLM “understood” which structural features correlate with degradation, the team prompted the model to generate new sequences that could induce even stronger effects.
But generating novel enzymes is just the beginning: the enzymes must work in reality, not just a computer. Two additional PLMs—one that ranks enzymatic activity, and one that predicts how and where the enzymes bind PFAS—help vet the enzymes generated by the first PLM. They believe that this approach (and subsequent finetuning and testing) will give them enzymes with the highest chance of binding and degrading PFAS.
In order for their synbio schema to succeed, the EXPLORE team’s PLMs must share data among themselves in a continuously improving feedback loop. To coordinate their PLMs in such an efficient fashion, they’ve called upon Lourenço’s own lab at Caltech, where a scientist is dedicating his own research to making PLMs accessible to scientists of all disciplines.
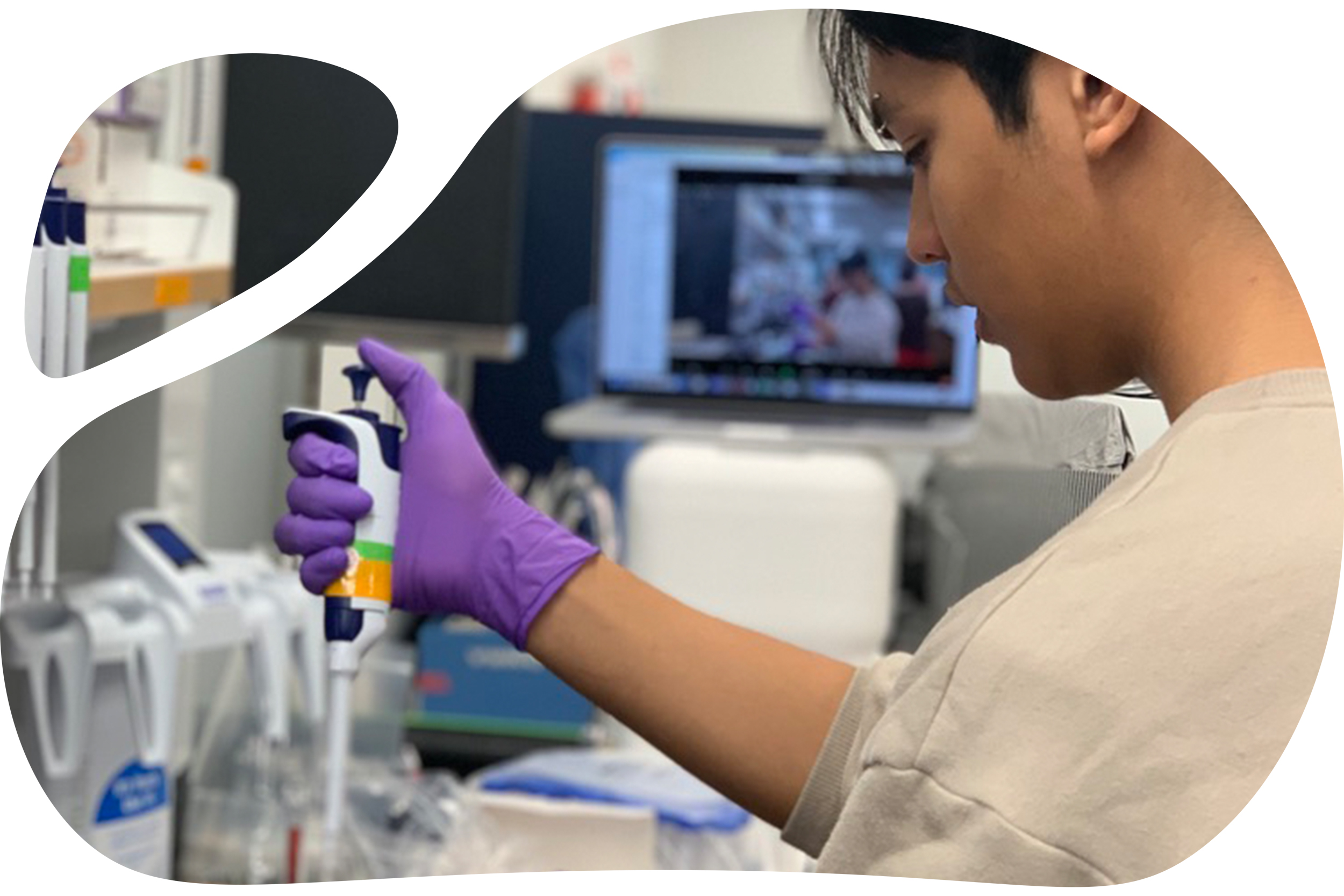
The Grammar of Life
The EXPLORE externship program is organized by the Institute for Educational Advancement (IEA), a Pasadena-based non-profit organization that challenges students who display advanced cognitive and socioemotional capacities.
The research is student-led and Monica Barsever, EXPLORE advisor and former high school science teacher, says that her EXPLORE cohort is teaching her just as much as she’s teaching them. Today’s teenagers will be among the first generation of scientists to enter the workforce already machine learning-savvy.
“As a scientist who hasn’t used machine learning, it has been super helpful for me to participate with them. And I think it would be great for other scientists to have that experience,” said Barsever.
Biology-specific generative AI tools are a relatively recent invention. In 2021, Google’s DeepMind released AlphaFold, a deep learning model that predicts protein structure. AlphaFold’s popularity among biomedical researchers gave rise to more PLMs that could predict other protein properties, like binding capacities or the movement of atoms within a protein structure.
Despite their intended applications in biological research, many PLMs are only accessible to coders. Zachary Martinez, a bioengineering PhD student at Caltech, realized that many biologists are unable to scale this barrier to entry.
“It seems like every time I talk to someone that isn’t from the machine learning space, they always have some biological application where they’d like to try to use these models,” said Martinez. “But I often get something along the lines of, ‘We tried to install the program, but it was such a headache.’ It’s just too high of an activation energy for most people.”
To make PLMs more accessible, Martinez and colleagues developed TRILL (TRaining and Inference Using the Language of Life), a publicly available suite of 20 preexisting PLMs and generative AI programs that create protein visualizations, generate protein structures from pretrained models, and predict protein properties. TRILL enables users to finetune these models for specific applications and feed data from one model into another, all within a streamlined command-line interface.
Accessible features like command-line interfaces make it easier for people without machine learning backgrounds, like the EXPLORE students, to access PLMs.
“You don’t have to be a software developer to work with the models in TRILL. It’s more accessible for people whose full-time job is biology or proteomics, and not coding,” said Lourenço.
PLMs have billions of parameters and are too large for most personal computers’ processing units. Only people with access to behemoth computing capacities—like engineers at big tech companies that developed PLMs in the first place—have the hardware space to house these models.
“One of the things I don’t think we appreciate is how expensive these models are to train,” said Martinez.
As a result, PLM applications rarely stray outside of drug discovery, a high-reward venture reserved for companies that can rake in the capital to employ these expensive and unwieldy tools. But researchers in less lucrative fields want in on the PLM party, too.
Postdoctoral researcher Dhan Fortela was one of the first people to apply protein language models to the PFAS problem. A chemical engineering instructor at the University of Louisiana at Lafayette, Fortela uses AI to advance research in sustainable energy and waste treatment. He sees environmental health as part of a triple Venn diagram overlapping with human health and machine learning.
“I don’t think that AI is scary. I try to think of it as a way to extend what exists,” said EXPLORE team member Lucas Garcia.
Fortela saw potential for DiffDock, one of the PLMs in TRILL’s suite, to solve problems outside of drug discovery. Developed in 2023 by researchers at the Massachusetts Institute of Technology, DiffDock predicts how drugs interact with proteins in the human body (or, the proteins of drug targets like bacteria or viruses). If DiffDock can successfully screen interactions between small molecules and proteins in a pharmaceutical context, why not apply that same technology to environmental health?
“A ligand is a ligand, whether it’s a drug molecule or a PFA,” Fortela said.
In a 2023 proof-of-concept study, Fortela and colleagues showed that DiffDock predicts the mechanisms by which PFAS could dock, or bind, to human blood proteins. Surprisingly, the study debunked previous claims that PFOS, one of the six PFAS affected by the EPA’s federal drinking water mandate, binds strongly with albumin. DiffDock’s diffusion-based model showed that PFOS actually binds with capillary blood glucose, a protein with similar properties to albumin. Fortela hypothesized that, in the studies that purported strong binding between PFOS and albumin, capillary blood glucose and albumin were likely co-extracted during sample prep in the lab. Machine learning models like DiffDock circumvent excessive sample manipulation that can lead to false positives or other inaccurate measurements. Nevertheless, Fortela believes that machine learning can enhance the quality of empirical measurements.
“It’s not the intent of computational work to challenge traditional techniques,” said Fortela. He explained that DiffDock, like other predictive AI tools, could not exist without large datasets of empirical measurements.
DiffDock was trained on empirical data from over 17,000 protein-ligand pairs. Thorough familiarization with the scope and nuances of data generated in real life helps the model make precise and accurate in silico predictions. “If there’s one thing that computational advancements are implying, it’s that we should be making more and better empirical measurements in the lab,” said Fortela.
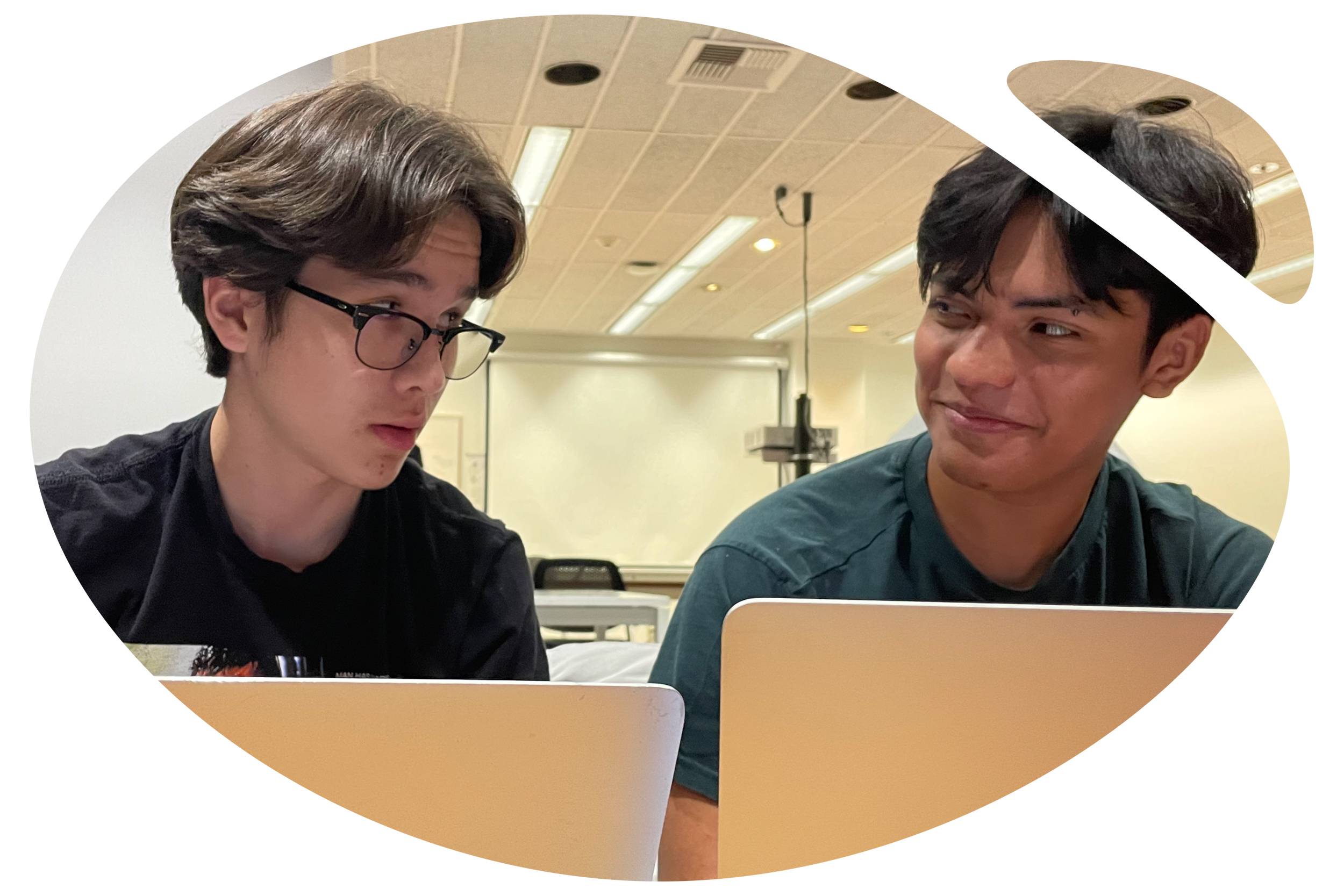
Scaling the Synbio Landscape
Lourenço agrees that PLMs work best in tandem with wet lab experiments. Even the best scientists have limited expertise and lab time—machine learning can expand the scope of their research. Lourenço equates PLMs’ expansion power to exploring the world by plane rather than limiting yourself to local roads.
“The way I like to think about it is, imagine you’re trying to find the biggest mountain in the world. If you’re in North America, you’ll go to Denali. That might be a good starting point, but you’re fundamentally limited by the characteristics of that mountain. By moving farther into the landscape, you can go to Africa and find Kilimanjaro, and go to Nepal and find Everest,” said Lourenço.
The EXPLORE team has already seen some success using TRILL and other PLM tools to scan the protein landscape. Surprisingly, their initial search returned several dozen natural enzymes with the ability, at least in theory, to degrade PFAS.
“We used this tool called FoldSeek, which is like a plagiarism checker for proteins,” said Dante Dam, a former team member. “It searches publicly available databases for proteins with structures and sequences similar to your input protein.”
The EXPLORE team fed FoldSeek a partial sequence from an enzyme experimentally proven to degrade PFOA into shorter-chain PFAS. FoldSeek returned nearly 70 natural enzymes with structures similar to the input enzyme. Structural similarity implies functional similarity, which means that these enzymes should be able to degrade PFAS to some extent. These proteins become more novel with each cycle of PLM finetuning, allowing the team to explore hundreds of ways that an artificial protein could bind and destroy PFAS.
“In our case, we want to get farther away from natural structures because we want to explore novel sequences that might be more efficient,” said Lucas Garcia in a YouTube presentation outlining the EXPLORE team’s project.
Garcia explains that there aren’t any existing PLMs that can accurately predict if an artificial protein could actually be produced in the lab. So, the EXPLORE team took matters into their own hands and started building their own AI model to predict which novel sequences would be the easiest to synthesize.
Just as the PLMs in TRILL feed data from one model into the next, the EXPLORE students build off each other’s specialized work to design a product that’s stronger than the sum of its parts.
Lourenço sees the ease with which his students question the status quo and adapt accordingly when it changes. He hopes that biotech companies, often prone to setting experimental boundaries too rigidly and too soon, can learn from his students’ approach to scientific research. He sees parallels between his students’ thought processes and the way that machine learning models “learn” from experimental boundaries, and hopes that these fresh perspectives encourage others to make synbio an even more inclusive and multidisciplinary space.
“We attach a label to scientists, which is that you have to be a straight-laced, by-the-book type of person. And that’s not really the case. Scientists are people too. Scientists experiment. Scientists have fun. And you can be a scientist, too,” Lourenço said.